Downscaled Soil Moisture Data
Downscaled Soil Moisture Active Passive (SMAP) @ 1-km Resolution via Machine Learning
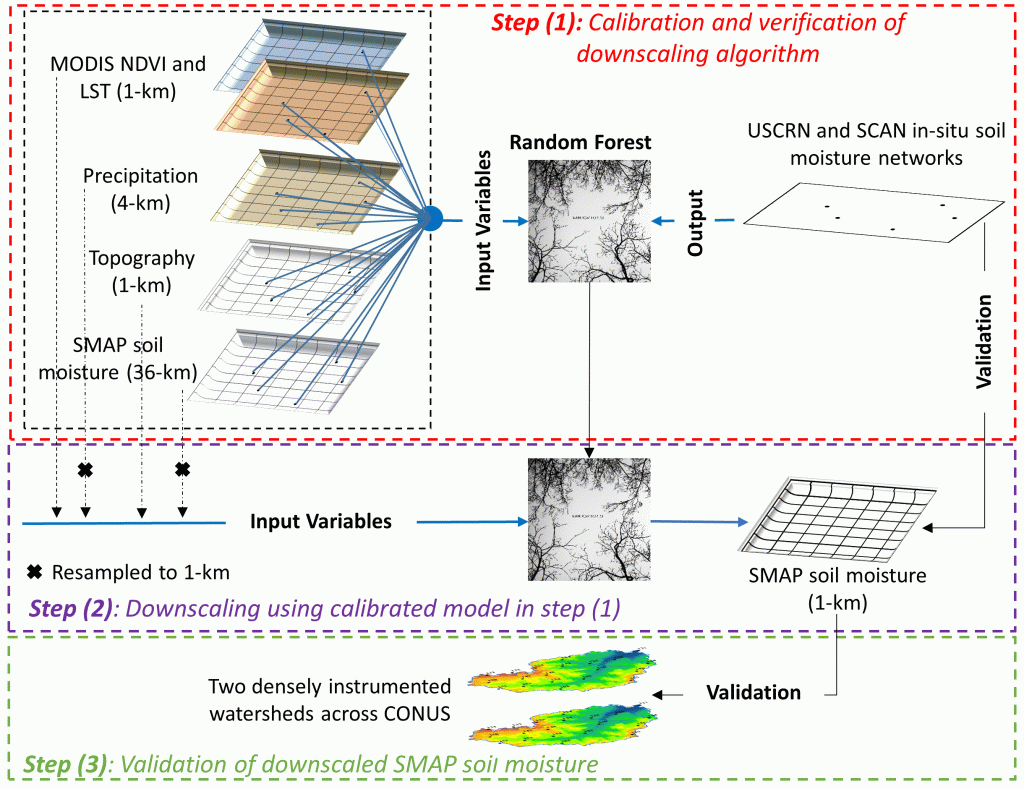
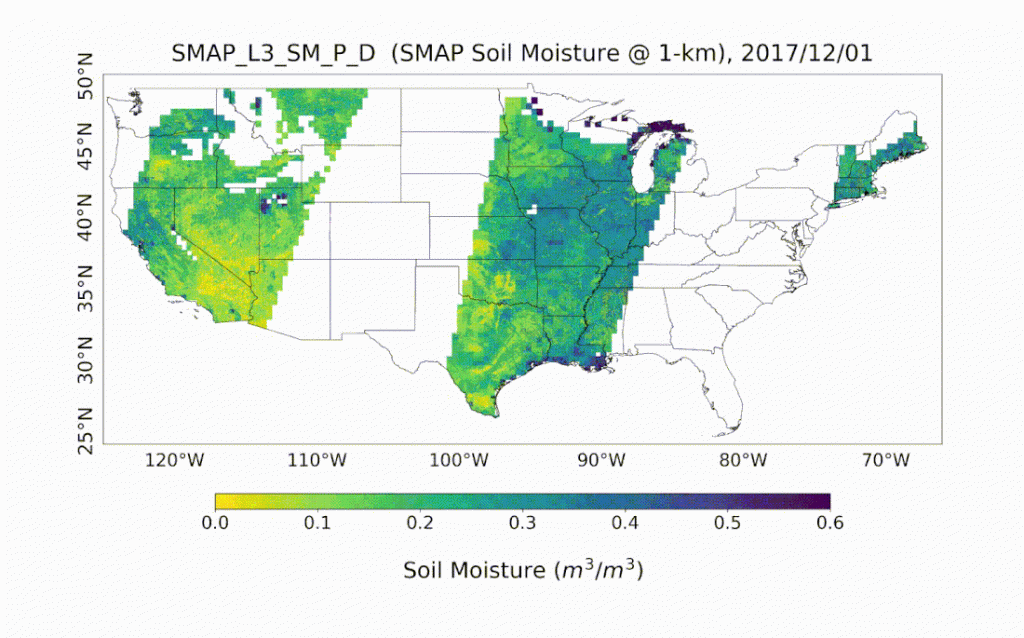
Remotely sensed soil moisture satellite data provide an unprecedented information about the soil moisture across spatial and temporal scales, which is logistically unachievable from in situ observation networks. SMAP (Soil Moisture Active Passive) is one of those satellites that provides soil moisture data at top 5 cm soil layer using brightness temperature on the 36‐km grid cell from both ascending (6:00 pm) and descending (6:00 am) passes. Although the SMAP soil moisture observation has a decent spatial resolution for global and continental scale applications, it cannot be used directly for regional or local studies, such as agriculture and drought monitoring, irrigation management and planning, flood forecasting, crop production and water resources management.
Here, we developed a framework based on machine learning and a suit of remotely sensed data (i.e., MODIS NDVI and land surface temperature and precipitation) and in situ data sets, including soil texture and topography data, to downscale the level 3 daily composite version (L3_SM_P) of SMAP radiometer soil moisture over the Continental United States (CONUS) to 1‐km spatial resolution, pivotal for a variety of applications. The downscaled soil moisture product (SMAP_L3_SM_P_D) has been validated against two core validation sites and over 300 sparse in situ soil moisture sensors evenly distributed over the CONUS.
References:
- Abbaszadeh, P., H. Moradkhani, X. Zhan (2019), Downscaling SMAP Radiometer Soil Moisture over the CONUS Using an Ensemble Learning Method, Water Resources Research, 54. doi:10.1029/2018WR023354.
- Abbaszadeh, P., H. Moradkhani, K. Gavahai, S. Kumar, C. Hain, X. Zhan, Q. Duan, C. Peters-Lidard, and M. Karimiziarani (2021) High-Resolution SMAP Satellite Soil Moisture Product: Exploring the Opportunities, Bulletin of the American Meteorological Society, doi: 10.1175/BAMS-D-21-0016.1
